Researchers at the Aachen Graphene & 2D Materials Center have released an open-source platform to automatically identify and classify exfoliated flakes of two-dimensional (2D) materials on a substrate, shortening one of the most time-consuming and tedious tasks in the study of 2D materials.
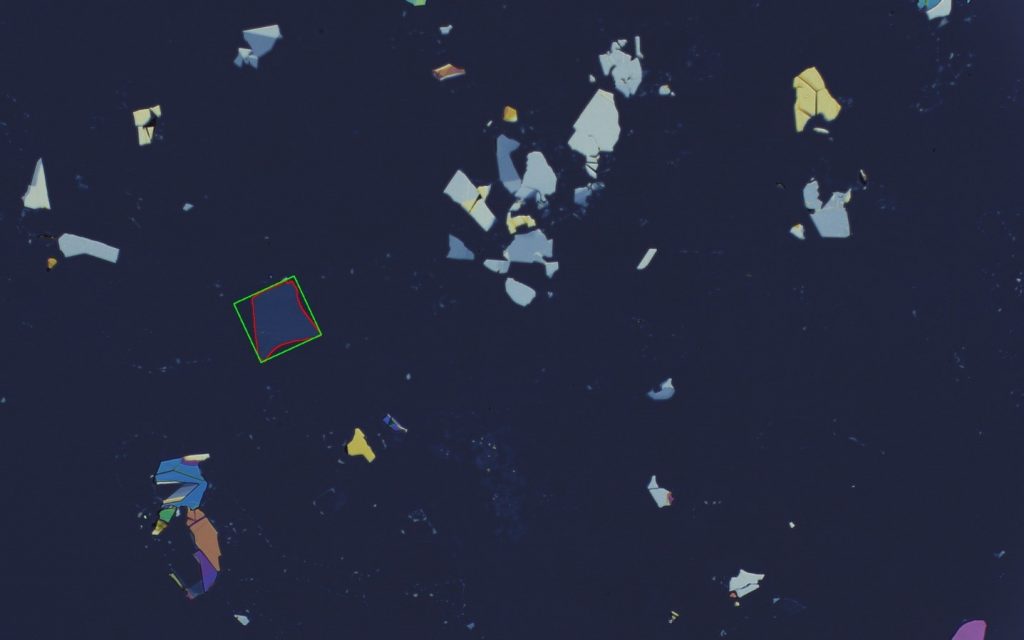